Introducing new technology propels a business the same way stomping the gas pedal propels a car. Whichever direction the car is already going, it’s now going that way faster. It’s an analogy grocers would do well to keep in mind as they evaluate the wide variety of AI-powered solutions proliferating the market.
AI is anticipated to revolutionize processes from ordering and forecasting to pricing and promoting. As such, it’s a hot topic many want to quickly implement to gain a competitive advantage. But what sometimes gets lost in all the excitement is a critical buzzword: machine learning.
What does the machine learn from? It learns from a company’s existing data. That’s why a grocer’s first step in evaluating new technology must be assessing the data and systems with which that technology will interact. Bypassing this foundational work inevitably leads to an underwhelming return on the time and money invested.
For example, consider a grocer that wants to decrease shrink, so it implements automated ordering technology. The grocer expects suggested orders and greater visibility into inventory levels will reduce overordering. But if the grocer hasn’t segmented its stores by purchasing patterns and optimized assortments accordingly, its stores are routinely stocking items that don’t sell. The automated ordering technology won’t fix this problem; conversely, it will scale it. Stores will continue ordering the wrong items, just faster and more consistently. Shrink persists.
Seeing the spectrum
A grocer doesn’t need a world-class data infrastructure to take advantage of the latest AI advancements, but it does need a clear view of its current data capability to understand how much value it can expect to extract from any new technology. To return to the earlier example, ordering more efficiently may save employees time and perhaps even help with retention if the job becomes much easier. However, shrink won’t decrease to the extent desired unless the grocer has the data and tools—and willingness to use them—to first optimize assortment. The grocer may still procure some value from a speedier process, but ROI will be severely limited.
Figure 1 provides a few examples of the information and tools grocers have at their disposal at varying levels of data capability (download the graphic as a PDF here):
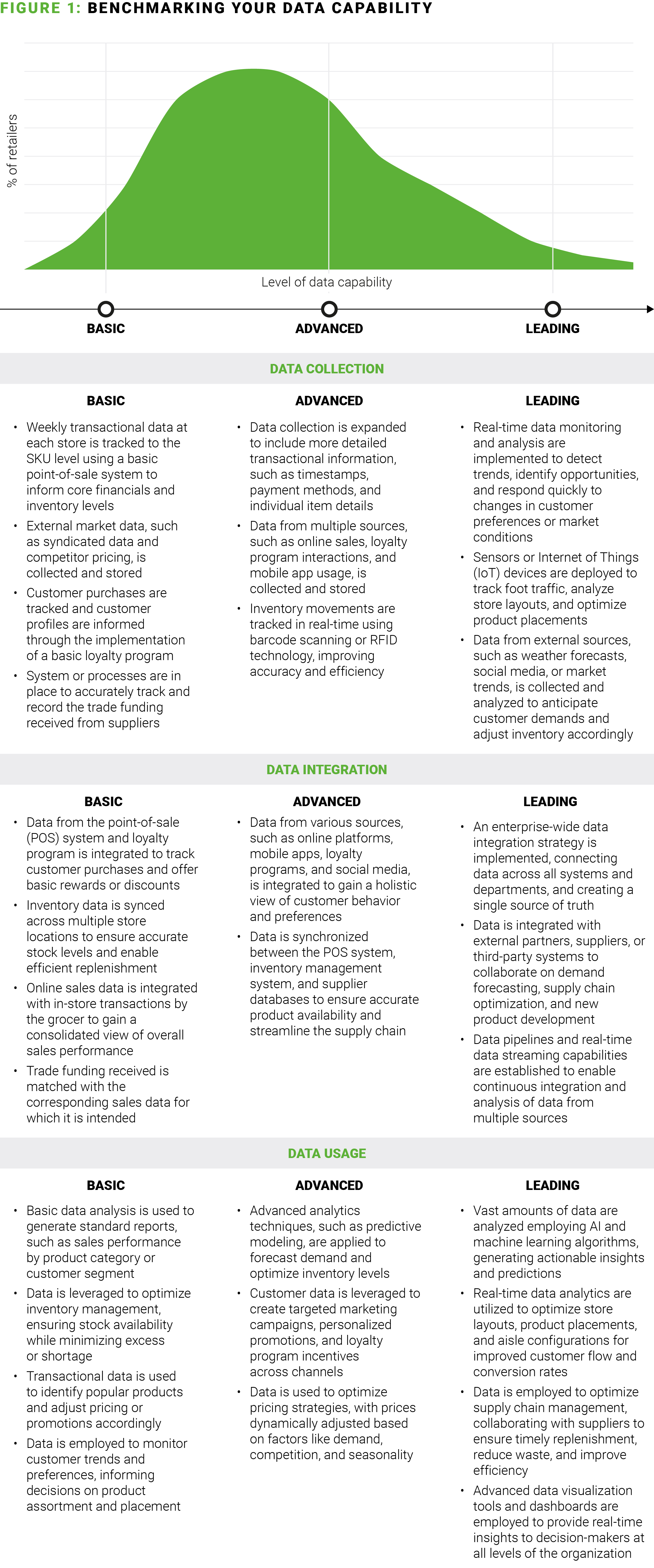
Data due diligence
Grocers evaluating their existing data infrastructure should consider five components:
- Raw data: It’s difficult to overstate the importance of complete, accurate data. With subpar data, grocers will have significant blind spots when they attempt to evaluate promotions, track competitor pricing, monitor raw material costs, leverage loyalty programs, and otherwise understand how the business is performing and where opportunities exist for growth.
- Data storage: Imagine a grocer has one system for tracking where trade funding is coming in, one system for tracking distribution, and one system that stitches together third-party data with the grocer’s POS data—but the systems aren’t connected. Integration is an important factor to consider when evaluating data infrastructure because it plays a significant role in the level of analysis you can conduct.
- Reporting tools for buyers: The usefulness of data is always limited by the format in which it’s delivered to the people actually using it. Grocers can’t expect their buyers to turn into data analysts overnight. Tools that provide suggestions, highlight priorities and risk, and run pricing simulations are game-changing.
- Reporting tools for strategic planning: Along with playing a role in buying decisions, advanced data tools help grocers rebalance across categories to influence customer perceptions and meet financial goals. Tools that assist in investment planning and optimization can help grocers evaluate how different levers—promotions, pricing, assortment, space planning, adjustments by store cluster, and more—contribute to desired outcomes.
- Analytics: Many grocers don’t have data analytics capabilities, but those that do have an advantage. They can study in detail the effects of their promotions—uplift, cannibalization, halo, and more—to understand price elasticities at category and sub-category levels. They can then segment their stores by how price-sensitive customers are and adjust accordingly. They can also rank SKUs by space contribution and sales, and compare raw material cost trends with supplier costs over time.
Foundation for the future
The next grocer to begin a deep dive on its data infrastructure and quickly discover a quagmire won’t be the first. Given the thin margins on which the business operates, data investments are often reactive rather than proactive. Decisions tend to focus on solving for an immediate need versus creating a comprehensive system designed to holistically contribute to the business.
Upgrading in the data arena doesn’t always mean starting from scratch. The level of data capability a grocer should pursue depends on what the goals are for the next five to 10 years—including the extent to which the grocer wants to take advantage of AI and other new technologies.
Want more? Check out these other recent insights from the grocery experts at AlixPartners:
Three ways grocery retailers can promote more profitably in fresh
The $100 billion opportunity for U.S. grocers
The grocery industry is ready to get back to basics … but there’s debate over what that means